Deployment of systems of Artificial Intelligence (AI) has become mainstream and popular with small and large economic operators. One of the most raging developments includes services relying on natural language processing (NLP) taking the form of chatbots.
In a previous blog post, I explored the opportunities the Digital Markets Act (DMA) provides the regulator to expand its list of core platform services (CPS) to accommodate generative AI as a service. Stemming from the initial discussion and thoughts triggered by that piece, I started reflecting on the dimensions of the interplay between generative AI and DMA.
As a result, I wrote a longer working paper presenting three distinct scenarios of how generative AI may be interpreted in the context of the regulation. Aside from the more obvious choice to include generative AI as a CPS, the European Commission may reach out for two tools from the toolbox: i) the application of the existing obligations to the underlying functionalities of the captured CPSs relying on generative AI; and ii) the designation of additional services from the existing list of CPSs.
This blog post focuses on those scenarios as a means to capture the phenomenon of generative AI by establishing the risks posed by each stage of the technology’s development process.
The underlying technology and the scope of the risks posed by it
Generative AI is as broad a topic as there can be. As opposed to other AI systems, generative AI systems create novel outputs. That is, they ‘generate’ novel outputs of text, images or other formats based on a user’s prompt. LLMs are a sub-category of generative AI, insofar as they are the text-based species of generative AI.
Problems in terms of the competitive dynamics generated by AI systems come into play under different lights and regarding distinct aspects of the market. The easiest way to depict them is to break down a generative model’s technical development into three stages as Figure 1 below: pre-training, fine-tuning and deployment.
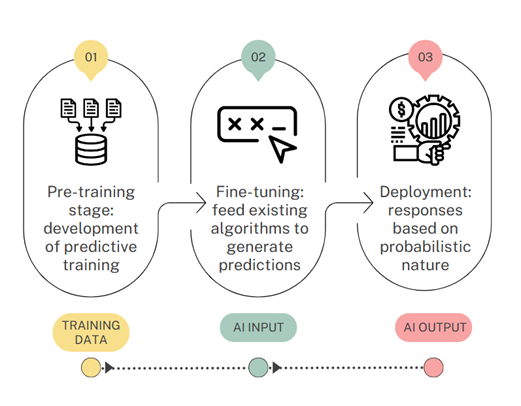
The capabilities of a generative model depend on a cluster of resources available at each of the three stages outlined in Figure 1 above.
First, they rely on training data so that they can be pre-trained. That is, generative models feed on huge troves of unstructured textual data. The model (the M in LLM) acquires a significant amount of general knowledge from diverse sources, including websites, books and articles. The model captures the nuances of grammar, syntax and semantics to be able, at the deployment stage, to predict the most probable combination of words it should generate in response to a prompt.
Once it is deployed, an LLM’s future performance improvement depends on both the computing and data sources the deployer can leverage at the pre-training stage. This preparatory phase may represent a bottleneck to the market since accessing such data is costly and its availability remains limited. Fixed costs are high whereas marginal costs remain low in comparison. Such a massive collection of data poses grave consequences in terms of data protection and privacy. At the pre-training level, the most simplistic consequence derives from a lack of transparency relating to the data sources and types the AI deployers access to conduct the task. Aside from that, substantial compute and energy cost goes into training LLMs.
Pre-training continues until the model achieves satisfactory performance on the task. In other words, until it successfully learns the meaningful representations involved in language. Despite holding the core knowledge of language, pre-trained models are not specialised enough to conduct any sort of specialised task. This is precisely the role of the fine-tuning phase. Fine-tuning input collections of specific examples stored in smaller and domain-specific datasets (input data) to enhance the model’s performance on specific tasks. Deployers start to tailor the kind of inferences they wish to display on the model at the deployment stage.
One of the most salient risks arising in the context of the fine-tuning stage is that of black box algorithms. The designers of such models have little control over the inferences generated as a result, due to the size of the training data and the complexity of the process. The very nature of self-learning AI operates with unknown variables and autonomous inferences, which oppose the data protection principles of both transparency and purpose limitation.
Once both pre-training and fine-tuning are completed, deployment does not necessarily entail launching the service into the market. In fact, deployers must place safeguards when launching their LLMs into production bearing in mind security and privacy concerns to achieve cost-effective performance. Distribution may take place via a standalone application (e.g., ChatGPT) or through integrations into existing applications and services (e.g., Apple’s release of Apple Intelligence into its devices).
AI outputs are inherently selective. The generative model provides a single synthesised answer in response to a prompt. Building upon the lack of transparency of the training data and the black box approach drawn into inferences, outputs may not reflect a direct response to a prompt but rather steer the user to select the most advantageous option to the deployer operating the model.
Capturing generative AI under the DMA (and not adding an additional CPS)
The most obvious policy option to capture generative AI under the DMA is to add another CPS to the existing list under Article 2(2). That is, perceiving generative AI as a standalone service meriting designation of its own, detached from other CPS categories. The European Parliament and the High-Level Group of the DMA have both hinted at this idea as a potentially satisfactory solution to counter the DMA’s regulatory cap. As I set out in the previous blog post I wrote on the topic, the legislative reform to make that incorporation possible would entail, at its very least, a 12-month market investigation followed by a legislative proposal drafted by the European Commission. That legislative proposal would then make its way to the European Parliament and the Council. The EU institutions would then decide whether such an amendment should be included in the DMA’s current legal text.
Generative AI as embedded functionality
Let’s say that we wish to speed things up. The European Commission has already designated twenty-four core platform services for seven gatekeepers. As pointed out by the High-Level Group, generative AI functionalities have already seeped into the DMA’s ambit of application to the extent that gatekeepers have embedded AI systems into their CPSs. Aside from their proprietary foundation models, gatekeepers such as Alphabet, Amazon, Apple, ByteDance, Meta and Microsoft have integrated generative AI-driven functionality into their existing products.
Functionality has been made available directly to the gatekeeper’s services. For instance, Google Search provides beta access in the US to its Search Generative Experience where generative AI integrates with its legacy service. The self-preferencing prohibition under Article 6(5) DMA does not exclusively apply to online search engines. Instead, it projects its effects on ‘ranking, related indexing and crawling’. Ranking in the sense of Article 2(22) DMA refers to the relative prominence given to goods or services or search results offered through social networks, virtual assistants, search engines or online intermediation services.
The nature of generative AI models and their outputs are purely selective, insofar as they choose to produce an answer from a set of alternatives based on their statistical likelihood, regardless of their truthfulness, accuracy or fairness. In the traditional sense, ranking happens based on the functioning and the underlying back-end functions of indexing and crawling, relying on an algorithm. The integration of generative AI into ranking entails the transformation of such an online experience. Going back to the example of Google Search, the interface of the search results changes. A single answer is displayed at the top of the page. The ranking at the top of the page does not correspond with the most relevant result in general but to a conversational response based on facts that the LLM extracts from publicly available data.
The transparency, fairness and non-discriminatory conditions that must apply to ranking as set out by Article 6(5) DMA impact the ‘enhanced’ versions of these services by generative AI. Considering the legal and technical challenges arising at the pre-training, fine-tuning and deployment stages, the DMA’s enforcer will have to carefully determine what transparency means in the regulation’s context.
Generative AI as technology
As opposed to considering generative AI as a standalone service, it can also be regarded as the underlying technology targeted to cater to existing functionality, which is in line with the principle of technological neutrality. Instead of adding a CPS to the existing list in Article 2(2), three categories of CPSs may capture the phenomenon (cloud computing services, virtual assistants and online search engines).
Cloud computing services are digital services enabling access to a scalable and elastic pool of shareable computing resources. The designation of cloud computing services addresses the top layer of the AI stack. That is to say, computing power scarcity, at least on the side of cloud computing providers. Since hardware-based GPUs are basically in the hands of one single player (i.e., NVIDIA), cloud computing stands as an alternative to access computing. Cloud infrastructure firms such as Amazon Web Services (AWS), Google Cloud, and Microsoft Azure are the three main operators of the market, and they have raised concerns with competition authorities regarding the market’s concentration. The oligopolistic-like market structure justifies their designation, albeit some authors argue the market does not display a particular lack of contestability, as required by the DMA.
Against this framework, the EC’s potential designation of cloud computing providers would only address the problem of access to compute power, which is not specific to generative models. At the outset, designating a gatekeeper for its cloud computing services does not add much to the generally applicable framework to the regulatory target, since no DMA provision is particularly tailored to address the concerns arising from that market.
Moreover, both virtual assistants and online search engines bear the nature of ‘qualified’ CPSs in the DMA, given that several mandates only apply to them, to the exclusion of any other CPS category.
For the online search engine CPS category, Articles 6(11) and 6(12) specifically address the need for the gatekeepers to provide access, on fair, reasonable and non-discriminatory terms, to two distinct tenets of its services. Article 6(11) mandates such access with respect to its ranking, query, click and view data, whereas Article 6(12) imposes this type of access on gatekeepers for the benefit of business users. Chatbots and the forms they adopt at the downstream level for searching capabilities could be considered services falling into this CPS category. Some firms exclusively cater for the search functionality via a standalone offer, such as Perplexity’s AI tool seeking to compete with Google’s search engine, whereas others have simply integrated search functions into their existing interfaces, notably OpenAI’s rolling out of ChatGPT Search.
In their definition under the DMA, search engines are digital services allowing users to input queries in order to perform searches of, in principle, all websites, or all websites in a particular language, on the basis of a query on any subject in the form of a keyword, voice request, phrase or other input, and returns results in any format in which information related to the requested content can be found. The definition’s main characteristic is that of intermediating queries (end users) with results (indexed results of business users).
Examples such as Perplexity’s service evidence that the deployment of generative models may result in equivalent services to those of online search engines in the sense of the DMA. Perplexity does not work in the same way as Google Search does. The main difference between them is that the former summarises search results and then produces source citations on its response, whereas the latter simply presents information as a list of the most relevant results. On top of that, Perplexity uses data on the consumer’s previous queries to provide personalised search results in the future. However, these features do not impact the chatbot’s purpose: to provide indexed search results in an accurate manner based on the user’s prompt. This situation would make for peculiar regulatory intervention since both Articles 6(11) and 6(12) would be applicable and the enforcer would have to interpret the criteria on fairness, reasonableness and non-discrimination in the context of the deployment of generative models.
For the particular case of virtual assistants, industry players across the board integrate ChatGPT plugins into their services so that consumers can have a conversational experience while the generative model performs basic tasks on their devices. Article 2(12) defines virtual assistants as software processing demands, tasks or questions, including those based on audio, visual, written input, gestures or motions, and that, based on those demands, tasks or questions, provides access to other services or controls connected to physical devices.
The EU legislature’s intentions in the context of the DMA are clearer here than they were when defining cloud computing services or online search engines. The last phrase of the definition (‘provides access to other services or controls connected physical devices’) hints at the idea that the legislator was not thinking of chatbots when regulating, but rather of the interconnectedness between Big Tech virtual assistants and IoT devices. To the extent that an impact on the user’s physicality is required, chatbots acting as virtual assistants do not yet satisfy the legal requirements to be considered virtual assistants in the sense of the DMA.
Key takeaways
Generative models bring different risks and tensions with the existing legal framework. At times, those challenges merit their capture through existing regulation. The DMA is not an inscrutable piece of regulation that does not allow for other legal alternatives if they are not directly contemplated in the letter of the law. On the contrary, the regulation’s interpretation demonstrates to be permeable in light of the current technical developments arising in the field of generative AI.
The enforcer can simply pivot towards different enforcement priorities by focusing on and understanding generative AI features as embedded functionality within the existing and designated gatekeeper’s services. Such an approach allows for the application of a wide range of DMA provisions to the particular needs of generative AI, namely the principles of transparency and fairness.
Likewise, those enforcement priorities may entail the triggering of additional CPS designations with respect to deployers of generative AI systems seeking to enter the markets of existing CPS categories, namely search engines or virtual assistants. In line with the principle of technological neutrality, generative AI may be regarded as a technology underlying the existing functionalities on the Internet, despite its technical complexity and particular nuances. As opposed to the first scenario, this perspective accommodates the current provisions to moderate gatekeeper behaviour stemming from non-designated undertakings.
To the European Parliament’s and Commission’s call to capture generative AI via the DMA, I would recommend applying a more intensive interpretative exercise on the regulation’s provisions. Such an approach may be more fruitful and targeted to meet the few evident contestability and fairness concerns arising from the lack of transparency of generative models.
________________________
To make sure you do not miss out on regular updates from the Kluwer Competition Law Blog, please subscribe here.
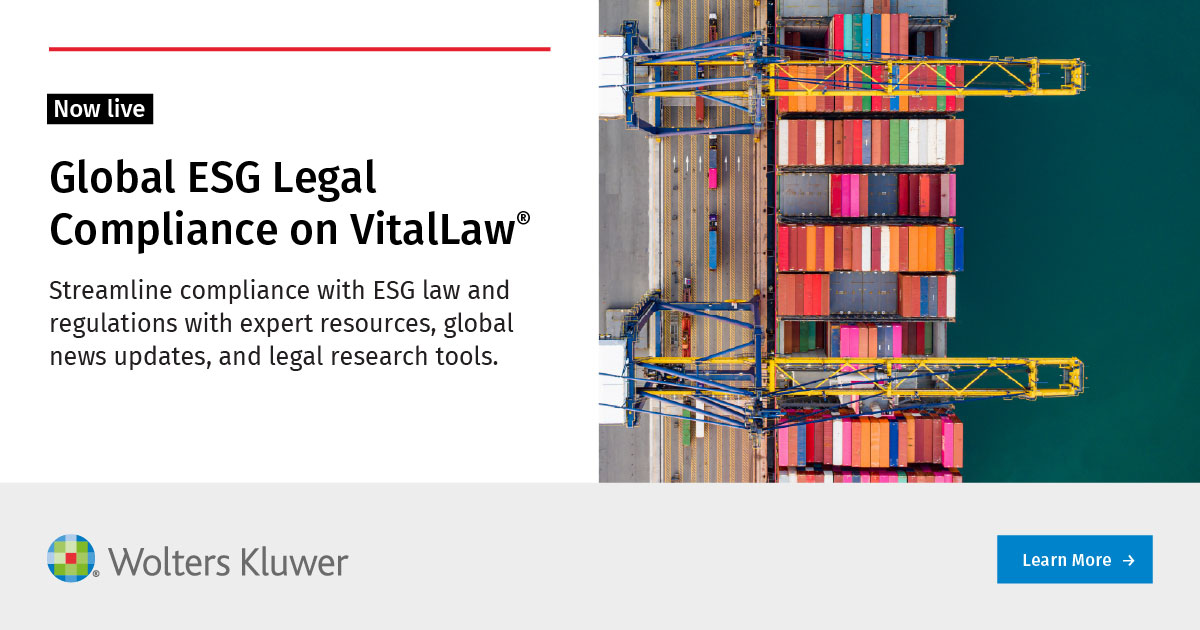
