Foundation models are not new to society or technological development. The deep neural network and self-supervised learning they are based on have existed for decades. However, the explosion and ramping up in user adoption of apps based on Large Language Models (LLMs) working on natural language processing (NLP) has shaken the whole tech industry during 2023. Stories of success can easily be spotted from Open AI’s launching of ChatGPT or the release (albeit sometimes only available in the US) of multimodal AI systems such as Google’s Gemini or Microsoft’s Copilot AI.
Even though the Digital Markets Act (DMA) was not initially intended to apply to LLMs and generative AI, the European Commission has already been asked to measure the intensity and degree of their interplay. The European Parliament has already called to include them, and the High-Level Group of the DMA also discussed the impact of their inclusion into the regulatory framework.
This blog post considers the overlap between both, bearing in mind two fundamental ideas. First, whether the DMA already applies to some foundation models, without the need to take recourse to legislative intervention. Second, whether the inclusion of foundation models into the list of core platform services (CPSs) under Article 2(2) DMA is sensible in terms of policymaking against the background of the existing provisions embroidered under the DMA and the safeguards already in place that will become applicable in the coming years as a result of the newly passed AI Act.
The comedy of errors: the conflated terminology of AI systems
Prior to exploring both questions, it is worth noting that the concepts surrounding AI systems are not straightforward. Several concepts are lightly used both in the press and by regulators. Thus, clarification is much needed if one attempts to provide more answers than questions when discussing the topic.
Foundation models serve as the bedrock upon which AI systems are built. They are trained on broad data and can be adapted to a wide range of downstream tasks (for a description of foundation models’ opportunities and risks, see here). This is why they are sometimes addressed as general-purpose AI because they provide the foundation for other AI applications. The AI Act directly adds provisions for providers of general-purpose AI, notably concerning those that pose a systemic risk based on its capabilities (an AI’s capability is normally measured against the benchmark of its computing power). Some models are termed as multimodal, which is not the same thing as saying that they are general purpose. Foundation models work with multiple data types, not only language, despite the fact that the narrative around powerful large language models (LLMs) has flooded the discourse around AI. The greatest of advancements was produced in language since most foundation models have taken shape based on the latest developments in natural language processing (NLP). In turn, when the foundation model draws from other modes of data besides language, for instance, vision data, it is said to be multimodal.
Two main elements characterise a foundation model: homogenisation and emergence. Homogenisation prompts the idea of the consolidation (concentration, perhaps?) of methodologies for building AI systems across a wide range of applications. For instance, all state-of-the-art NLP models are now built from one of a few foundation models. This element poses the risk that the same inherent biases of a few foundation models may plague their downstream application (a range of scholars have already denounced this circumstance here and here). Emergence means that the system’s behaviour is implicitly induced rather than explicitly constructed. Therefore, there is no need for more bespoke feature engineering pipelines. In turn, this characteristic creates substantial uncertainty over foundation models’ underlying capabilities and flaws. Paired with the homogenisation feature, emergence may generate a scenario where a few foundation models may entail great risk for societies and markets.
Generative models (aka generative AI) are a type of foundation model that generates original content by deriving patterns and relationships within the data and its context which is trained from existing content and translated into text. All generative models are foundation models but not the other way around because the former are tailored to serve specific tasks with less versatility than foundation models.
What are the current stakes of the game?
The real-world deployment of AI systems entails far-reaching consequences on people and the competitive dynamics of markets. These models are used in two fundamental ways: to bring new products to the market and to upgrade the existing products and services with new capabilities. The launch of Google’s LLM Bard is a good example of the former, whereas Google Search’s dependence on language models like BERT and MUM for improving its search results is exemplary for the latter. From the European point of view, LLMs are also taking the market by storm, such as (French) Mistral AI, based on open-source software. The social impact caused by the permeation of foundation models (or their downstream applications) into the market spans from fairness considerations to potential concerns of amplifying disinformation.
However, when one considers the DMA’s subject matter, policymakers have placed two substantive risks at the top of their priorities. First, foundation models are trained on data that is selected using unspecified or unclear principles with a general lack of transparency regarding the nature of training data. The overlap with the GDPR’s provisions is evident. For example, it may well be the case that a foundation model trains on special categories of personal data that are, pre-emptively, prohibited from being processed under EU data protection regulation unless the requirements under Article 9(2) GDPR are fulfilled (see Jo’s and Gebru’s analysis of the clash in detail). From the perspective of intellectual property, there is the argument to be made that general-purpose models should not be able to extract and train on data behind paywalls (for the discussion, see the recent New York Times lawsuit against OpenAI and Microsoft). The counterargument is, from an IP perspective, that the training process can benefit from an exception or limitation to copyright rules such as in text and data mining or even, where it is applicable, the fair use doctrine (see more here).
Moreover, the fact that foundation models train on massive troves of data under the banner ‘the more data, the better’ (see Kaplan et al. on the relationship between a model’s efficiency and the data embedded within it) begs the question of whether the functioning of these models is at odds with the principles of data minimisation, storage limitation, and purpose limitation under the terms of Article 5(1) GDPR.
The DMA is particularly concerned with the leveraging of data performed by gatekeepers across one area of activity to another, especially when these same undertakings exercise control over whole ecosystems which are structurally and extremely difficult to challenge or contest by existing or new market operators (Recital 3 of the DMA). Several provisions are carved out against this same blueprint, namely Articles 5(2), 6(2) or 6(10) DMA.
Second, the absolute cost of computation to develop a foundation model only is within reach of a few institutions and organisations. The analogy goes that the economics of foundation models entail setting money on fire (see here). The players that have access to these relevant computational resources and the massive troves of training data will likely determine who can produce cutting-edge foundation models. This circumstance raises potential concerns about market concentration since a few players operate as enablers to grant access to the capacity to develop these foundation models. Bottlenecks are, translated into the terms of the DMA, important gateways for business users to reach end users, which is one of the main characteristics that makes an undertaking an addressee to the regulation (as per Article 3(1)(b) DMA).
In the face of both risks, one can argue that the intersection between foundation models, generative AI and the DMA is absolutely required to ensure consistency and coherence in policymaking and to future-proof effective enforcement of the regulatory instrument. In this regard, two types of solutions are presented before oneself: addressing the risks in contestability by applying those data-driven provisions under the regulatory framework to foundation models (at least, those of the designated gatekeepers) or triggering a legislative development to explicitly introduce foundation models into the DMA’s scope of application via their listing as a CPS.
The current interplay between foundation models and the DMA
The relationship between the current regulatory framework embedded in the DMA and generative AI is not completely evident. Scholars have advocated that both fields remain distinct and, thus, the DMA does not adequately cover generative AI systems, regardless of the fact that integration is advisable (despite that predominantly traditional theories of harm have already been analysed under the lens of generative AI, see here). This argument is contested. Another group of academics sustains that several provisions under the DMA will significantly affect the development and deployment of AI by gatekeepers and their competitors.
The DMA applies to the designated gatekeepers and the core platform services that are directly delineated within the designation decisions issued by the European Commission in September 2023 (see a comment on those decisions here). At face value, not one of the designation decisions issued by the European Commission mentions that a CPS within the gatekeepers’ services relying on generative or foundation models is included under the scope of application of the DMA. The EC was not reluctant to include under the DMA’s scope of application services that did not abide by one of the categories of CPSs listed in Article 2(2) DMA. For example, it is included under Meta’s WhatsApp service, its Business Application Programming API, despite the fact that APIs are not directly listed as a CPS (see Meta’s designation decision, para 130). This demonstrates that the fact that foundation or generative models are not listed as CPSs is, preliminarily, not an obstacle to their appraisal under the DMA’s terms. Notwithstanding, the first designation decisions issued by the Commission entirely skipped the matter.
In pushing the limits of this same argument, one could derive from the EC’s designation decisions that they enclose much more than what simply catches the eye. Even though foundation/generative models are not directly referenced, the EC’s designation decisions could be interpreted to enclose an implicit reference to them and, thus, they would entirely fall under the scope of application of the regulatory framework. For instance, if Google Search is one of Alphabet’s designated CPSs, and they must be understood bearing in mind a technologically neutral approach (under the terms presented in Recital 14 DMA), then the search results underlying technology should also be captured under the regulatory instrument, e.g., the powering of the service through MUM to provide more targeted results. A similar example may apply once Meta’s Instagram introduces its AI-powered image editing which will depend on generative models.
If that were to be the case, then the EC would have to apply the substantive provisions of the DMA to generative AI applications. For the data-driven provisions, this circumstance would not lead to any substantial difference, given that they are mainly concerned with data within a CPS being leveraged onto another CPS of the gatekeeper’s or its first-party services. Despite the fact that the underlying AI-powered technology of Google Search influences the advantage that Alphabet obtains in the market in the form of search results, the mandates borne into the DMA do not prohibit data processing or combining within the same CPS. Thus, the regulatory framework’s discontinuation of a gatekeeper’s leveraging strategy to stop them from tipping the market via the feeding off personal and non-personal data, would not directly touch upon the AI-powered functionalities of the CPS.
The implicit appraisal of generative models would make more sense for those provisions that relate to the legislator’s concerns around fairness. For example, the application of the prohibition of self-preferencing would be more advisable, since the conditions in which MUM intervenes over the results that are displayed on Google Search would have to abide by the transparent, fair, and non-discriminatory benchmark placed by the provision. In other words, the generative model should be fine-tuned and adapted according to the parameters engrained within the underlying technology. Those fairness parameters would crystallise, in practice, into methods for technical mitigation centred on data and the modelling decisions involved which, in turn, may target different steps in the pipeline. This may prove particularly challenging if the generative model is a downstream application of the foundation model that lies outside of the hands of the application developer.
Aside from contestability and fairness considerations, the DMA considers the fulfilment of the objective of transparency via opening the information disclosed to advertisers and publishers when they are supplied online advertising services provided by the gatekeeper (Articles 5(9) and 5(10) DMA) and providing them with access to the performance measuring tools of the gatekeeper to carry out their independent verification of advertisements inventory (Article 6(8) DMA). Article 15 DMA also entails that the gatekeeper shall disclose through an audit the techniques for profiling consumers that the gatekeeper applied to or across its CPSs. These provisions tackle the need to verify the gatekeeper’s data operations and AI-powered operations that happen ‘under the hood’. Within this context, generative AI tools would entirely fall within the scope of the obligations given that they are not defined through their relationship with other CPSs, but in relation to their impact on the decisions they make in the market and in relation to consumer profiling.
Grounds for including generative models as a CPS into the DMA
Deriving from the complex terminology around AI systems, it is worth noting that the impact of the inclusion of an additional CPS into the list under Article 2(2) DMA will depend on the type of service included. The same conclusions will not derive from the fact that general-purpose AI is included as a CPS as opposed to the introduction of generative models. For the sake of simplicity, the analysis is centred on the inclusion of generative models to the list under Article 2(2) DMA.
The regulatory instrument lists ten different services as core platform services under Article 2(2) DMA. A definition of CPSs is not elucidated throughout the DMA, although their presence is necessary to designate an undertaking as a gatekeeper. The legislator, however, does prompt at the fact that those CPSs were included in the DMA’s scope of application due to their characteristics, which can be exploited by the undertakings providing them. For example, such characteristics include extreme scale economies, very strong network effects, the multi-sidedness of their services, the significant degree of dependence produced on both business users and end users, lock-in effects, or vertical integration (Recital 2 of the DMA). The trove of CPSs included in the DMA is not stagnant. To ensure that the regulatory instrument remains up to date and constitutes an effective and holistic regulatory response to the problems posed by gatekeepers, the European Commission can expand and eliminate services from the lists of CPSs included in Article 2(2), according to the terms presented in Recital 77.
The EC cannot simply adopt a decision to include a service as a CPS in the DMA. Article 19 DMA requires the public authority to conduct a market investigation to examine whether one or more services within the digital sector should be added to the list laid down in Article 2(2) DMA. In the assessment performed within the market investigation, the EC shall take into account any relevant findings of proceedings under Articles 101 and 102 TFEU concerning digital markets, as well as any other relevant developments. The resulting report from the market investigation will be passed on to the European Parliament and the Council. If the new service is to be included as CPS, a legislative proposal will be accompanied by the report presenting the necessary amendments. Despite the fact that the provision does not directly reference it, the threshold that the EC must surpass to reach this last step of the way is that of reaching a solid evidentiary basis that supports the amendment (Recital 77).
The procedural safeguards around the inclusion of another CPS into the DMA are not scarce and trivial, due to the heavy burden of intervention that designation entails for any given undertaking that is designated as a gatekeeper. The threshold of a solid evidentiary basis is not that evident for the EC to overcome, especially in light of innovative digital sectors that are still emerging. Additionally, it also is not entirely apparent what factors the EC would consider for conducting the market investigation, aside from observing the degree of concentration at the industry level and the practices that may hint at the presence of unfair practices in the sector. The previous analysis of the configuration of generative models does not make it completely obvious that although those tendencies to data accumulation and homogenisation prevail, they might be successfully captured via the DMA’s mandates and prohibitions.
In this context, the most immediate example of an undertaking that the EC would desire to capture under the scope of the DMA would be that of OpenAI. Under the assumption that generative models are included by a legislative amendment to the regulatory framework, then its designation would follow through. However, that does not necessarily imply that the inclusion of generative models into the list under Article 2(2) DMA comes without friction in relation to the prohibitions that are engrained into it. The most salient provisions would be particularly at odds with the business models of generative AI, especially those relating to the processing and combining of personal and non-personal data across the CPS’s services.
Key takeaways
Theoretically, generative models and the DMA fundamentally intersect in relation to the characteristic of homogenisation. Computation power in a few hands can pre-empt the configuration of a few bottlenecks influencing the operations of the rest of the competitors at the level of the downstream application of foundation models into concrete tasks and functions. Aside from this salient element, how both subjects may interact in reality is not straightforward:
- If one assumes that they already interact with relation to those CPSs that have already been designated that possess AI-powered tools, the application of the DMA’s substantive provisions is not completely evident, albeit those provisions are directed at the objective of enhancing transparency.
- If one undertakes the path of the inclusion of generative models under the list of CPSs under Article 2(2), friction still prevails, since the mandates of the DMA are evidently at odds with the fundamental functioning of generative and foundation models, notably their training, adaptation, and modulation.
Against this background, the EU’s precautionary principle should spearhead the whole discussion on the interplay of generative AI with the DMA’s provisions, by awaiting the solid evidentiary basis that sustains the conclusion that markets have tipped in favour of a few bottlenecks. The European Commission’s call for contributions regarding the competitive dynamics of generative AI has gotten a good head start towards this same point. Once there is sufficient scientific evidence on the issue, then the legislator should seriously consider what are the chances of restoring the competitive conditions in generative AI through antitrust-like remedies in the face of this idiosyncratic, changing, and complex sector.
________________________
To make sure you do not miss out on regular updates from the Kluwer Competition Law Blog, please subscribe here.
Kluwer Competition Law
The 2022 Future Ready Lawyer survey showed that 79% of lawyers are coping with increased volume & complexity of information. Kluwer Competition Law enables you to make more informed decisions, more quickly from every preferred location. Are you, as a competition lawyer, ready for the future?
Learn how Kluwer Competition Law can support you.
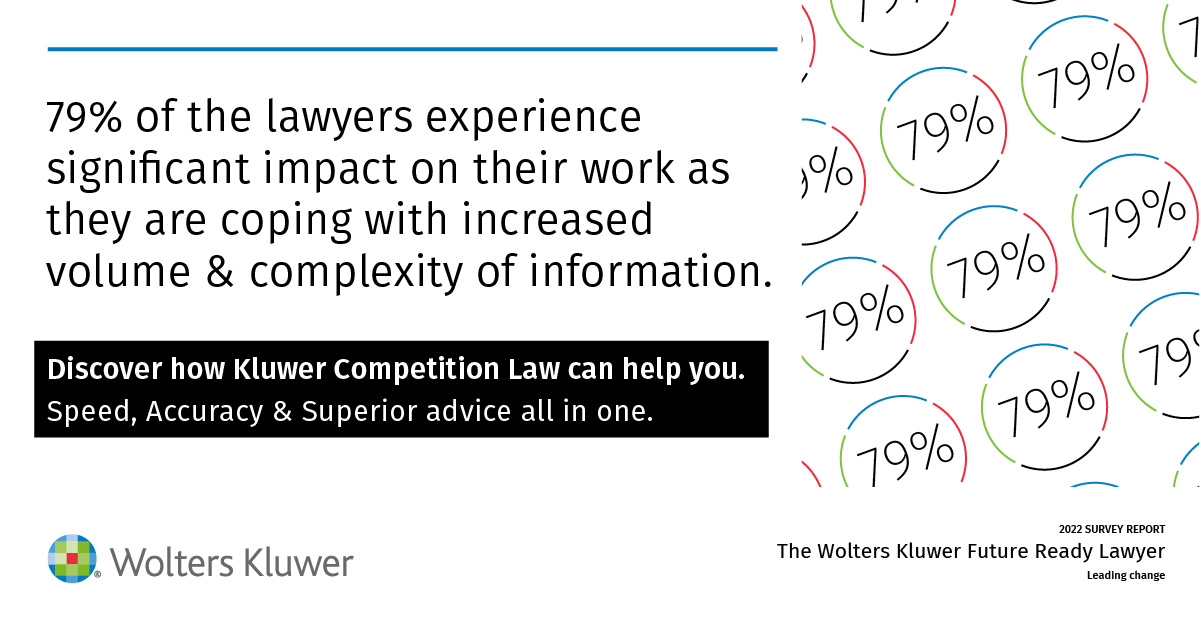
