On 14 April 2025, Meta confirmed that it will start training its AI with publicly available data from Europeans so its models “can understand the incredible and diverse nuances and complexities that make up European communities”. Regardless of the wider repercussions of such a move from the data protection perspective, since Facebook, Instagram, WhatsApp and Messenger were designated in September 2023 as core platform services (CPSs) under the DMA, there is an unavoidable link to trace from the policy to its impact with the European regulation addressing market power.
As I commented on a previous post, although generative AI is not listed in the DMA as a CPS, generative AI functionalities can be captured via the regulation, to the extent that they are embedded in already designated services. For instance, Google’s new search engine generative experience via AI Overviews exactly fits the meaning. In particular, the prohibition of performing personal data combinations across CPSs (Article 5(2) DMA) seems at a crossroads with the training and fine-tuning of generative AI functionalities owned and operated by gatekeepers. By building on my last working paper, the post presents the tenets and the consequences they bring to AI deployers.
Personal data used for training an AI model
Generative AI is a sub-species of general-purpose AI models. Normally, AI models generate content based on a set of instructions inputted by the user (prompt). LLMs specifically focus on language modelling to generate content, whereas multi-modal LLMs merge an AI model’s capacity to generate text alongside other forms of outputs, such as video or image generation. In the LLM space, state-of-the-art generative AI models include OpenAI’s ChatGPT, relying on its latest GPT4, Google’s Gemini 1.0 Ultra, Anthropic’s Claude 3.7 Sonnet, High-Flyer’s DeepSeek or xAI’s Grok-3.
Content generation through AI stems from the model’s learning of patterns and characteristics from large unstructured datasets. Based on transformer architecture, the model does not learn how to speak a particular language. It statistically grasps a broader understanding of language by tokenising strings of words and symbols and then attributing them with weights based on their importance. As a result, the generative AI model imitates the patterns that one uses to speak language with those words that are more likely to follow the preceding ones.
To reach these learning and generative capabilities, large-scale AI models interact with data of all kinds in two fundamental ways: they feed and process large datasets at the pre-training and fine-tuning stages, and they interact with the data inputted by users via the prompts they insert into the technology. Although AI deployers sometimes collect training data via consensual extraction and data sharing, billions of tokens built into popular LLMs derive from web scraping. Scraping refers to the retrieval of content available online via automated tools. Normally, scraping takes place over publicly accessible websites, including, for instance, social media profiles. Technically, paywalled websites can also be subject to scraping.
In the particular case of the training of LLMs, Common Crawl, the largest freely available collection of ‘scraped’ data (with more than 9.5 petabytes of data ranging from 2008), constitutes one of the most relevant sources of pre-training data for LLMs. Nowadays, most AI deployers use Common Crawl as a data baseline and then train their models on a variety of filtered versions from it. On top of that, inference attack methods have been applied to existing state-of-the-art LLMs and demonstrated that they trained on paywalled websites, copyrighted content, and books, also scraped from the web. Paywalled news sites ranked top in the data sources included in Google’s C4 database (used to train Meta’s LLaMA and Google’s LLM T5).
Evidence on copyright or privacy infringements takes the form of a piecemeal approach, whereas AI deployers oppose transparency when documenting and disclosing the training sources by which their LLMs have been optimised. Thus, one cannot simply assert that this or that LLM was trained on a dataset where personal data and/or personal information was stored, nor can one rule the possibility out completely. Uncertainty in this field poses broader questions of law, notably in the areas of privacy, data protection, and intellectual property.
The prohibition under Article 5(2) DMA and the tensions with the GDPR in the context of generative AI functionalities
The GDPR occupies a prominent role in determining the legal requirements that go into processing training data for generative AI. It is not, however, the only regulation to have a direct impact on such processing activities. The Digital Markets Act (DMA), in its current design and framework, poses fundamental challenges in this field as well, despite the apparent loud silence of regulators and AI deployers. The DMA applies to seven designated economic operators that have been designated by the European Commission (Alphabet, Apple, Amazon, ByteDance, Booking.com, Meta, and Microsoft). Thus, only these participants in the market will be subject to the limitations spelled out for AI deployers.
Building on the experience of cases surrounding Meta’s processing activities, the DMA introduces the prohibition embedded in Article 5(2) DMA. The provision compels the economic agents designated by the European Commission not to process, combine, or cross-use personal data from their services into other services, either first-party or third-party. Processing of personal data using services of third parties is only barred for those cases where it is performed to provide online advertising services.
By imposing the prohibition, the DMA seeks to end barriers to entry placed by the data accumulation capacity of these incumbent digital platforms. In practice, this means that, for instance, Meta cannot combine data for advertising across its services (Instagram, WhatsApp, Facebook, Facebook Marketplace, and Facebook Messenger) nor with other third-party services (e.g., other social network providers or advertisers). Likewise, Google cannot combine personal data across its services to feed its online advertising services, where it monetises much of its business.
Despite the prohibition and the DMA’s pledge to apply without prejudice to other pieces of regulation, such as the GDPR, the regulation exempts the prohibition in those cases where the end users concerned by the processing and combining of personal data consent to the activities. This is why incumbent digital platforms introduced a wide range of prompts to garner end user consent for this purpose once the DMA obligations started to kick in (see, for instance, Microsoft’s 2025 compliance report, pages 2-8).
On top of that, Article 5(2) provides an additional caveat to the prohibition: the conduct is also ‘without prejudice’ to the gatekeeper processing personal data relying on the legal basis set out in Article 6(1) GDPR (Recital 36 of the DMA). However, the legislator excludes the undertakings’ capacity to rely on the legal bases of Article 6(1)(b) and (f) GDPR. Those operators designated by the European Commission as subject to the DMA will no longer be able to rely on the legal bases of the data controller’s legitimate interest or of the necessity of the performance of a contract.
The exclusion of the legitimate bases for processing personal data based on the gatekeeper’s legitimate interest is not inconsequential. In fact, data protection authorities have defended that this is the only legal basis available to data controllers to perform tasks related to AI training and fine-tuning, under a set of exceptional circumstances.
In turn, the DMA paves the way for an unlikely scenario as far as generative AI is concerned. Following the prohibition under Article 5(2), incumbent digital players will be forced to silo the processing, combining and cross-using of their personal data per each model they integrate within their services. By this token, Gemini 2.0, which has been incorporated into Google’s search engine, should have only been trained and fine-tuned with personal data to perform data combinations in the search engine. Any other combination and cross-use would be pre-emptively prohibited by the DMA.
By taking the reasoning to its extreme, each model trained on personal data should be integrated into a different service, bearing in mind that they cannot be cross-contaminated due to the regulatory provision. Microsoft already responded in kind by ensuring the European Commission that its generative AI-reliant functionalities available on LinkedIn only feed on the service’s data. Anticipating possible backlash, it also declared that all fine-tuning and training of its LLMs excluded EEA-based members’ personal data (Microsoft’s LinkedIn compliance report, para 23). Microsoft, therefore, ensured that its LLM honours the user’s consent settings to run inferences on the model. Other captured economic operators by DMA remain keenly silent on such integrations.
Furthermore, the letter of law does not provide much workable guidance in terms of the legal bases the AI deployer can rely upon when training and fine-tuning its model, even in those cases where data protection frameworks are complied with. Bearing in mind that consent as a legal basis to train and fine-tune an AI model is rarely manageable, given the sheer amount of data subjects involved, one should, then, turn to the available legal bases under Article 6 GDPR. Article 5(2) establishes that “this paragraph is without prejudice to the possibility” for the captured agent to rely on some of the legal bases, whereas Recital 36 declares that “this should be without prejudice to the gatekeeper processing personal data or signing in end users to a service relying” on the available legal bases.
Although the consequences may not be quickly apparent to the naked eye, the prohibition brings grave repercussions to how an AI deployer combines and cross-uses personal data for training and fine-tuning purposes. Depending on the interpretation performed by the enforcer, such a prohibition, encompassing the limitations applied to the legal bases the deployer can access to process personal data, may bring grave consequences for AI gatekeeper deployers.
On one side of the spectrum, AI deployers categorised as regulated targets under the DMA would have no real possibilities of developing their own foundation models and applying them to downstream applications in the EU, as they do in other jurisdictions, since they would have no available legal bases to process personal data for the purposes of training and fine-tuning their AI models. In turn, the option open to them would be to outsource their generative AI-reliant functionalities. If they are not in charge of the AI model and, therefore, of its decision-making (and cannot be categorised as data controllers relating to the processing), then Article 5(2) DMA would have no bearing on them. Apple’s choice to integrate OpenAI’s ChatGPT and Alphabet’s Gemini into its Apple Intelligence feature on its operating systems is a good example of such a detachment from liability.
On the other hand, the enforcer may place the legislator’s choice of excluding some legal bases for processing personal data as a clear policy choice to elevate consent as the preferred means to exempt the prohibition. In other words, the granting of consent should be considered as primus inter pares in terms of its capacity to override the prohibition.
Accordingly, the remaining legal bases may come to the rescue to make the prohibition good in the eyes of the enforcer, except for legitimate interest and performance of a contract. As per the analyses of data protection authorities, in the absence of consent, legitimate interest is the most robust legal basis to rely on. Unaided by the legal support, data combinations, processing, and cross-use of personal data fall adrift of meeting the DMA’s regulatory standards. Thus, the prohibition persists and transversally applies to both the training and fine-tuning stages of AI development. Alternatives such as relying on the ‘outsourcing’ of foundation models or downgrading the model’s capabilities in the EU space still remain feasible policy options, despite the fact that the consequences of limiting the legitimate interest legal basis are less pronounced.
Key takeaways
Generative AI models rely on large-scale data processing, at times involving personal data sourced and powered by web scraping. Data protection frameworks and authorities have voiced their concerns about the lawfulness of such practices. Less attention has been devoted to the obligations that the incumbent digital players of the space bear insofar as they are captured as regulatory targets under the Digital Markets Act.
As opposed to streamlining processing, combining, and cross-using data as a result of web scraping, the DMA depicts a different scenario where the regulatory targets must rein in their training and fine-tuning tasks to first-party contexts and away from leveraging third-party data into their models. Crucially, the regulation also excludes these AI deployers from relying on the legitimate interest legal basis for the processing of personal data, albeit the scope of such limitation is not completely clear if one looks at the letter of the law. Bearing in mind that data protection authorities argue that consent might not be a feasible legal basis to rely on, the provision significantly affects the feasibility of training and fine-tuning generative AI models for these incumbent digital players.
________________________
To make sure you do not miss out on regular updates from the Kluwer Competition Law Blog, please subscribe here.
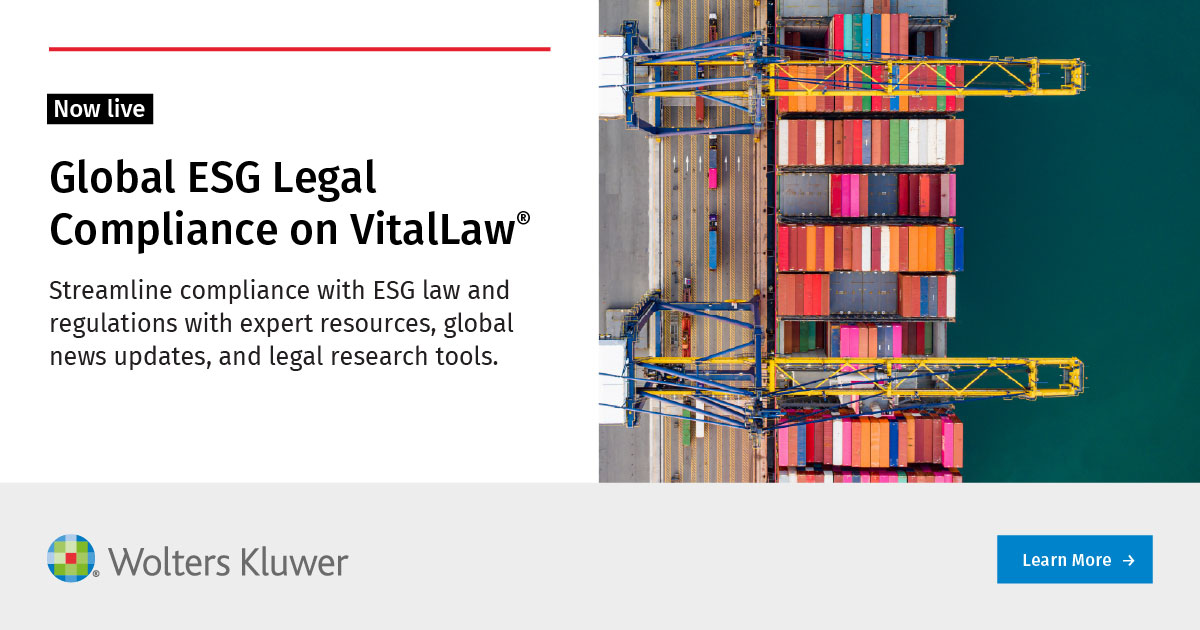

To what extent can data scientists ensure lawful use of large-scale training data without compromising the performance of generative AI models?